top of page
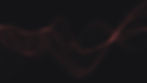
Lorem Ipsum
Papers

Lorem Ipsum
I'm a paragraph. Click here to add your own text and edit me. It’s easy. Just click “Edit Text” or double click me to add your own content and make changes to the font. I’m a great place for you to tell a story and let your users know a little more about you.
bottom of page